Solving on-shelf availability: Balancing accuracy, recall, precision & actionability
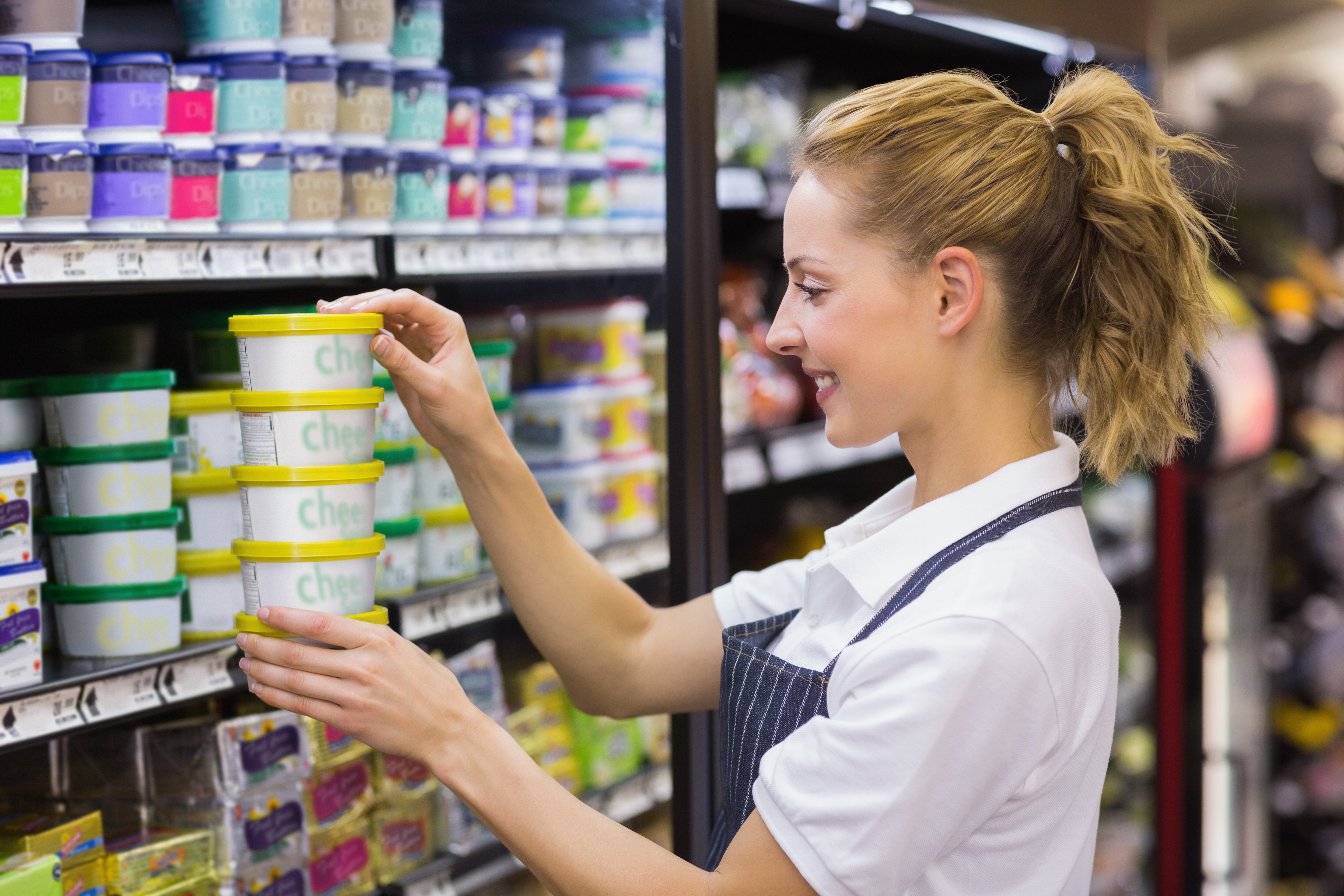
- 5 minute read
- Retail Insight Data Science Team
There are many retail analytics solutions that utilize advanced statistical models to generate intuitive output that can be acted on by head office and store colleagues to drive KPI performance improvement. It is imperative that these models are accurate to make optimal use of the constrained labor available in-store – inaccuracy simply wastes valuable time. But a singular focus on accuracy can also limit value creation – for example, the intervention may not be actionable, or an overly cautious model may fail to identify all the issues. Thus, in practice, we strive to maintain an optimal balance between model precision and recall, whilst maximizing actionability.
A focus on the terminology can be a little dry, so let’s discuss these terms in the context of real-life examples, using our AvailabilityInsight product – used to identify and prioritize out-of-stock (OOS) items. Accuracy relates to the number of correct OOS calls made by the product – were we right? Recall is the percentage of the total number of actual OOS occasions that occurred, how many of them were flagged by the availability product – did we catch them all? Then precision is the determination of how many of the flagged OOS calls were correct – were we right? Finally, actionability is the model’s conclusion, did the alert allow an associate to act upon it – was there a practical next step?
Example 1: Shelf out-of-stocks
One of the biggest challenges in retail operations is maintaining product availability. If goods are not available for customer purchase, then the retailer may lose out on sales. Among other things, our AvailabilityInsight model provides alerts to stores about items that are inferred to be OOS on the shelf. Each alert is either correct (i.e., the product was OOS) or incorrect (i.e., the product was not OOS – a ‘false alarm’).
For example, suppose our store has 100 items and AvailabilityInsight sends alerts for 10 of these as being OOS – meaning 90 of the items were predicted to be in-stock and available for sale. Of these 10 alerts, eight were OOS, meaning there were two false alarms. Then, of the 90 non-issues, one of them was incorrect – or a missed opportunity. Meaning there were actually nine OOS in total. In this example, this is how the terms stack up:
- Accuracy = (8 correct alerts + 89 correct non-issues) / (100 total calls) = 97%
- Precision = (8 correct alerts) / (10 total alerts of OOS) = 80%
- Recall = (8 correct alerts) / (9 actual OOS) = 89%
Actionability: will be determined by whether the correct alerts had accessible stock on hand for replenishment.
Together, we can see that simply saying “the model’s accuracy is 97%” does not tell the full story; recall was 89%, with precision at 80%. In addition to this, not all correct and incorrect calls are equal; some false alarms will be quick for associates to check, whilst the lost sales value of a missed call may be a very low revenue, low turnover item. In both instances, the total impact is far lower than it could have been.
Example 2: External field agent intervention
Brand owners, manufacturers, and suppliers often deploy teams of field sales agents who visit stores to, among many other things, check that their products are on the shelf and in the correct location and to ensure every sales opportunity is being achieved. To increase the effectiveness of these visits, many suppliers leverage our field sales tool which processes many data feeds to automatically identify those items that contribute most to the lost sales opportunity. Along with historical sales data, the model also incorporates a range of variables to help determine alert actionability at the time of visit.
In some cases, we can sacrifice the recall to attain a higher precision, as field sales teams only have a limited amount of time to check a limited amount of the total alerts. Selecting the desired threshold is complicated, as we need to ensure we are not sending too few, or too many alerts to any store. Additionally, we also do not want to waste time by sending alerts that the team cannot action.
So, let’s explore how precision and recall interact with each other.
Striking the right balance
In practice, achieving both 100% precision and recall is not possible. On one side, too precise a model will reap only a fraction of the potential benefits of identifying actual issues, as it avoids false alarms. Conversely, having an overly sensitive model will identify many more correct issues – but at the same time incorporate more false alarms. Neither position is optimal for our client; therefore, we look for a suitable trade-off between the two.
Our AvailabilityInsight product leverages a probabilistic model (e.g., 98% confidence that this product is not on the shelf), and by choosing a suitable probability cut-off, we can induce a suitable balance between precision and recall. To assist with this, our teams will use a precision-recall curve to show what level of precision and recall we would achieve at that cut-off. This enables us to visually see the trade-off directly in a single plot, facilitating the selection of our probability threshold.
Figure 1: Precision-Recall Curve
The graph illustrates the process we go through to design an appropriate model. Recall is on the x-axis and precision is on the y-axis. There are three corresponding probability thresholds included to demonstrate their links to the measures. As we move left to right, our probability threshold decreases as we become less cautious about which alerts we send to a store, our recall increases, and our precision decreases which results in more false alarms from the model.
In the current food retail market, there is a high cost to sending false alarms to stores as it wastes an already constrained labor resource on tasks that they cannot influence. Yet, during other times capturing every possible alert will be acceptable if the cost of not selling an item is so great. The correct balance between precision and recall will be determined by the balance between the benefit from correct alerts and the cost of incorrect ones.
In conclusion, no model will behave the same in every client. It demands regular tuning to ensure its outputs are optimized to achieve the maximum level of value for the client. This optimization will need to continuously occur because of numerous different factors; seasonality, store location, weather, etc. This is the distinct capability Retail Insight’s Decision Science team brings, we do not just have world-class mathematical knowledge, but we also have strong retail domain expertise that enables us to balance such complex trade-off problems across all our products.
Get in touch
Written by Retail Insight Data Science Team
Driven by strong retail domain expertise, the Data Science Team develops proprietary statistical and mathematical algorithms, based on first principles, in combination with advanced AI and ML, to deliver complex projects.